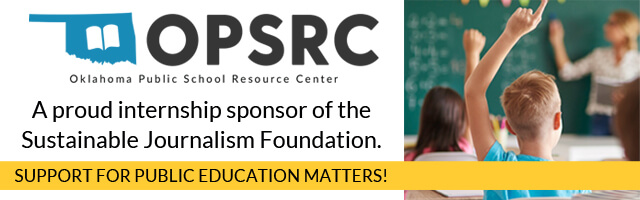
The wife of Noah Webster, he of dictionary fame, once came home to find her husband in bed with the maid. “Noah,” said Mrs. Webster, “I am surprised!”
“No, my dear,” Noah responded. “I am surprised. You are astonished.”
Astonished certainly described my state of mind Nov. 9 when I woke to find the results of the presidential election. I have voted in every presidential election since 1968, and while I have always considered the choices on their merits, I have always ended up choosing the Democrat. Never was that choice so clear as in 2016, and all the pre-election polls seemed to say that my fellow Americans in large majority agreed with me. Part of my astonishment was that the polls were so wrong.
At least in our shared astonishment, a supermajority of Americans agreed with me: The Gallup poll found that 69 percent were surprised [sic] at the outcome of the presidential election. Or to be precise — and to start a discussion of what the mathematics of polling really says — in a random sample of 1,000 interviewees (the thousand is a round number typical of a Gallup sample size), there were 690 who said they were surprised. The mathematics of polling uses this data to find lower and upper limits – with a 95 percent probability of being correct – for the proportion of surprised individuals in the population.
The 2016 presidential polls versus reality
The four major broadcast television networks (ABC, CBS, NBC and Fox) in conjunction with national newspapers (Washington Post, New York Times and Wall Street Journal) all released poll results based on data collected just before the election. I want to use that data to calculate the proportion of voters that intended to vote Clinton. The population I want to consider will be just those eligible voters who intended to vote for either Clinton or Trump. So in the samples I only consider those respondents who had one of those preferences. Also, assuming, as is necessary, that the four polls all met the requirement for random samples, we can combine all four to get one large random sample.
We end up with a random sample of size 5,750, of which 2,995 intended to vote Clinton and the remaining 2,755 intended to vote Trump. The proportion of interest is that of the Clinton voters. In this sample, the sample proportion is 52.09 percent. For a sample of size 5,750, and using the 95 percent criterion, the margin of error is 1.29 percent. Thus we conclude that there was a 95 percent probability that, of the population of eligible voters intending to vote for Clinton or Trump, the proportion who intended to vote for Clinton fell somewhere between 52.09 percent plus or minus 1.29 percent (53.38 percent and 50.8 percent, respectively). Note that even for the lowest limit, it’s still the case that Clinton was forecast to get slightly more than half the votes.
In reality (and as of the time of this article’s editing), there were 127,253,037 votes cast for Clinton or Trump. Of these, 64,777,890 (or 50.9 percent) were for Clinton, just barely above the lower end of the predicted range. In other words, this is one in the 95 percent of times that polling correctly predicted the actual result.
If we run the same numbers where the proportion of interest is Trump voters, then the sample proportion is 47.91 percent, with the same margin of error of 1.29 percent. Thus, the upper-limit estimate for the population proportion (percentage of Trump voters) is 47.91 percent plus 1.29 percent (49.2 percent), which was still less than half the votes.
Numbers in polling headlines exist within a range
News media, including those who commissioned the polls just analyzed, like to report the sample proportion as their headline number. There is nothing wrong with that, as long as their readers and viewers keep in mind that what is really being produced is an estimating range — and one that is only assumed reliable 95 percent of the time at that.
For example, suppose in the above sample that 140 fewer voters actually chose Clinton. Then the sample proportion (of Clinton voters) would have been 49.65 percent (not a majority). So the sample proportion in this scenario predicts a loss. The limits in this case are 49.65 percent plus or minus 1.29 percent (50.94 percent and 48.36 percent, respectively). The limits still capture the actual tally percentage of 50.9 percent, so such a sample is possible.
Any time the interval contains percentages both below and above 50 percent, the only conclusion we can fairly draw is that the data is inconclusive, even though the sample proportion would represent a theoretical loss or win.
The real world of polling
Actual polls are more complicated than any of the examples considered here, because real-world polls are trying to assess multiple proportions at once. The presidential polls, for instance, have to reckon with voters whose alternative to Clinton could be a third-party or write-in candidate, or even voters who intend to skip the ballot’s presidential section. The polls examined here are those commissioned by media to generate news stories that attract viewers and readers.
Political campaigns also do their own extensive polling to see how they are doing and where to direct resources. These polls, which are private, may reveal information the news media polls miss. Of course, the structure of presidential elections, particularly the Electoral College, means a much finer analysis than just national polls is required to predict the outcome.
As we saw, the polls’ prediction was consistent with the actual popular vote. That the sample proportions weren’t actually on the mark for predicting the electee has led to much post mortem analysis, lots of it based on exit polls, with the irony being the use of new polls to explain why previous polls failed to accurately predict a past result.
Finally, any gloom that remains about the failure of data analytics to predict the presidential winner needs to be tempered with the achievement of the Chicago Cubs, who, like the Boston Red Sox before them, managed to use statistical analyses known as sabermetrics to capture a long-elusive World Series.